DESIGN TEAM CONVERGENCE
Understanding the engineering design process from a cognitive perspective is highly valuable. The insights gained can shed light on ways to be more efficient and successful as design engineers, as well as to inform the building of computational tools to aid engineers during the design process. Most of the design that happens in industry today cannot be accomplished by a single designer. The increasing complexity of the products demanded by customers and the inability of any one design engineer to be an expert in all necessary fields requires that a team of designers be engaged. Thus, understanding the effects of external influences on the cognitive mechanisms employed by members of design teams is critical to understanding and improving the engineering design process.
This study was concerned with controllable external conditions that could stimulate or inhibit the production of better solutions in more efficient ways by any team of design engineers. The key question to be answered is:
"How is the convergence and quality of solutions produced by groups of engineering designers affected by the information they are initially given and, more specifically, by a poor example and good example solution?"
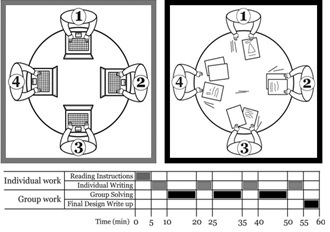
To understand convergence, a model of the team members' solution approach was developed through a cognitive engineering design study, specifically examining the effect of the introduction of a poor example solution or a good example solution prior to problem solving on the quality of the produced solutions. Latent Semantic Analysis (LSA) was used to track the teams' convergence, and the quality of design solutions was systematically assessed using pre-established criteria and multiple evaluators. Introducing a poor example solution was shown to decrease teams' convergence over time, as well as the quality of their design solution; introducing a good example solution did not produce a statistically significant different effect on convergence compared to the control (with no prior example solution provided), but did lead to higher quality solutions.
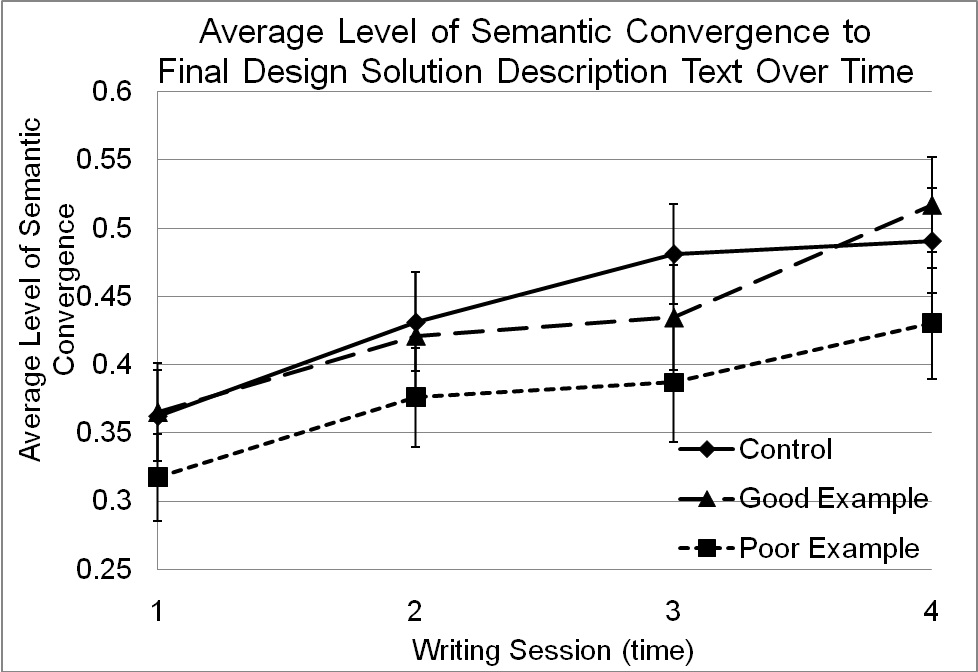
Primary Researcher: KATE FU
|